*Abdul Azim Muhammad Zulhilmi1, Yuichi S. Hayakawa2
(1.Graduate School of Environmental Science, Hokkaido University, Japan, 2.Faculty of Earth Environmental Science, Hokkaido University, Japan)
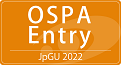
Keywords:Co-seismic, Landslide susceptibility, Machine learning, Landslide factors, 2018 Hokkaido Eastern Iburi Earthquake
The 2018 Hokkaido Eastern Iburi Earthquake caused large amounts of devastating landslides in the hillslopes in Atsuma and Abira towns. The landscape in this region is not extremely steep, but the co-seismic landslides dominated likely due to the lack of cohesion in the surface tephra layers. This research provides spatial insights into the pre- and post-earthquake landslide susceptibility environment in the Atsuma region. This high magnitude earthquake may influence adjacent faults for potential activation. Furthermore, external forces such as heavy rainfall combined with shorter intervals of weaker earthquakes could also cause slope deformations. Therefore, the potential of generating new landslides is a probability especially in the remaining slopes that tephra layers have remained intact. We aim to utilize machine learning algorithms such as random forest and logistic regression to demarcate new regions of susceptibility and possibly compare generated susceptibility maps to identify performing causal factors such as topographical, hydrological, and geological attributes that have a significant influence on these landslides. We also discuss the reasons of large-scale non-sliding areas that remained un-triggered during the earthquake event. The challenges of this projects include the translation of such scientific results for the local inhabitants. If communicated properly, such landslide susceptibility map could potentially aid in risk management and mitigation.