*Saori Nakashita1, Takeshi Enomoto2
(1.Graduate School of Science, Kyoto University, 2.Disaster Research Prevention Institute, Kyoto University)
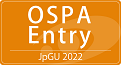
Keywords:Maximum Likelihood Ensemble Filter, Observation space localization, Nonlinear observation
We propose a new observation space localization method for the maximum likelihood ensemble filter (MLEF; Zupanski, 2005) that evaluates the nonlinearity of observation operators more appropriately than conventional ensemble Kalman filters and enables an efficient parallel implementation. The observation space localization in the local ensemble transform Kalman filter (LETKF; Hunt et al., 2007) uses observations within a local domain to make an analysis for each grid point. This is equivalent to evaluating the ensemble weights for each grid. Since the ensemble weights are calculated analytically, LETKF performs a local analysis independently for each grid, yielding high parallel efficiency. On the other hand, MLEF is an ensemble-variational method and calculates the ensemble weights by an iterative optimization of a nonlinear cost function. With MLEF, a local analysis may use a gradient defined at each grid but requires the predicted observations in the domain during optimization, hence entailing communications of the state vector in a parallel implementation. Previous studies show that the ensemble weights are spatially smoother than state variables (Yang et al., 2009, Kotsuki et al., 2020) . Our new method defines a local cost function for each grid under an assumption that the ensemble weights are constant in the local domain. This assumption allows independent optimization for each grid and makes this method more suitable for parallelization than the method that uses local gradients. In this study, we applied these two localization methods to MLEF, one with local gradients and the other with local cost functions, comparing them against LETKF in cycling data assimilation experiments with the Lorenz-96 model. Both types of localized MLEF produced more accurate analysis than LETKF when strongly nonlinear observations were assimilated. As the nonlinearity became stronger, LETKF converged slower during the assimilation cycle and varied larger in accuracy among trials. By contrast, the localized MLEFs were consistent regardless of the strength of the nonlinearity. Comparing the MLEF using local gradients and that using local cost functions, the latter showed better performance in the case of relatively weak nonlinearity. This difference is considered to be associated with the convergence of optimization. The ensemble weights had a high spatial correlation with those at neighbouring grids, which supports the assumption of constant weights. Our new method has an advantage on scalability over the one with local gradients. These encouraging results show that our new method is applicable to higher dimensional problems.