*Momoko Nakamura1, Yuji Nakamura1, Hiroki Uematsu1, Yukino Yazaki1, Ahyi Kim1
(1.Yokohama City University)
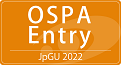
Once earthquake occurs, it is very important for disaster prevention to figure out the exact spatial distribution of seismic intensity as soon as possible. In order to achieve this, it would be ideal to install seismographs in high density, but this is difficult due to the human and economic costs. In reality, we should interpolate the intensity, but there are many issues such as the accuracy of geological information. To overcome this issue, deep learning-based seismic intensity prediction without geological information has been proposed and shown to be successful (Otake et al., 2020). In this study, we attempted to construct a real-time seismic intensity (Kunugi et. al, 2008, Ir) prediction model using long-short term memory (LSTM), one of deep learning architecture, in the vicinity of Yokohama City, which has much less training data than previous studies, and verified the performance of the model. In this study, following Otake et al. (2020), an existing K-net observation point was used as a prediction target, and the model was trained with data from four surrounding K-net observation points to predict the Ir of the target that was not used for training. In the previous study, they used stations in the Tohoku region, which allowed them to secure a large number of aftershocks from the 2011 off the Pacific coast of Tohoku earthquake, and the number of earthquakes used for training reached over 2,500. However, in this study, the number of earthquakes shared by all stations is 236 from 1996 to 2020, which is one tenth of previous study. As a preprocessing, the three-component seismic waveform of 60 seconds after the start of recording was converted to Ir and resampled to 100Hz. The model consists of a mask layer, three LSTM layers, a dropout layer that exists between the LSTM layers, and a total coupling layer. MSE was used as the loss function, and linear was used as the activation function for all coupled layers. The model trained in this study had 50 LSTM units, a batch size of 1000, a learning rate of 0.001, and was trained in epoch 20. We used 10% of the total data as test data, 10% of the remaining 90% as validation data, and 90% as teacher data.
The prediction accuracy was compared with the weighted average and the maximum input value. The weighted average was calculated using the distance from the forecast point to the input observation point as the weight. The maximum input value is a value that mimics the PLUM method, and Ir is calculated using the highest value among the four input observation points as the seismic intensity of the forecast point at each time. The MAE and RMSE values of the forecasted and observed values for the entire time series were 0.08 and 0.13, respectively, for the LSTM model. We also compared whether the LSTM model predicted the same values around the time when the actual real-time seismic intensity (observation Ir) took the maximum value. We compared the weighted average of the difference between the maximum predicted value and the observed Ir in the 0.5 seconds before and after the time when the observed Ir took the maximum value with the maximum input value. The mean of the differences was 0.065, which was the smallest for the maximum input value, and the standard error was 0.039, which was the smallest for the prediction of the LSTM model. These results indicate that the LSTM model is more accurate to predict the time series data itself, but the maximum input values are superior for the final seismic intensity. However, when we looked at the time trends in detail, we found that Ir predicted by LSTM had large fluctuations and often showed large instantaneous values, which greatly affected the estimation. This may be due to the fact that this study has less training data than Otake et al. (2020). In the future, we will re-evaluate this prediction model by adding a moving average and other smoothing measures and by increasing the number of stations to increase data.