*Seiya Fujishima1
(1.Department of Geology and Mineralogy, Graduate School of Science, Kyoto University)
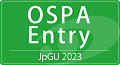
Keywords:turbidity current, turbidite, inverse analysis, machine learning, convolutional neural networks
Turbidity currents are gravity flows generated intermittently in the deep sea, driven by the density difference with the surrounding fluid. In-situ observations have revealed that various hazardous events, including tsunamis, trigger turbidity currents. Thus, turbidite may provide a clue to estimating the magnitude of past large tsunamis and paleo-earthquakes. Recently, a deep learning neural network (DNN) inversion model using a one-dimensional forward model has been developed to estimate the initial conditions of turbidity currents from their deposits. However, one-dimensional models of existing studies cannot adequately represent behaviors of turbidity currents because of the complex topography of sedimentary basins in the active margins, such as the Japan Trench, where tsunami-induced turbidites are deposited. Thus, developing an inverse model using a horizontal two-dimensional forward model is required. Here, the inverse model was developed using a convolutional neural network (CNN) employing a two-dimensional four-equation model of turbidity currents as the forward model in this study. The performance of the inverse model was then verified using the artificial test dataset and the results of flume experiments. The procedure for developing the CNN inverse model is as follows. First, training datasets were generated by repeating forward calculations under various initial conditions. Next, the CNN was trained to learn the relationship between the initial conditions and the characteristics of the deposits using the training datasets. The trained inverse model was finally utilized to estimate the hydraulic conditions of turbidity currents from the sediments. Verification of the inverse model performance using artificial test datasets implied that the inverse model in this study was able to estimate the initial conditions of turbidity currents from deposits with high accuracy. Then, flume experiments of turbidity currents were conducted in an experimental tank (2.2 x 4.5 x 1.5 m), and the inverse model was applied to the experimental deposits. The results of the inverse analysis showed good agreement between the measured values and the inverse analysis results for the depositional characteristics, whereas the reconstructed hydraulic properties of flows largely deviated from the measured values. Relative errors of estimation in the flow height were less than -86%, which is a relatively good estimation, but those for the suspended sediment concentrations were 143 times larger than the measured value in maximum. The fact that the sediment characteristics could be reconstructed suggests that the CNN inverse model’s ability to search for hydraulic conditions that reproduce sedimentary features is sufficiently high. Nevertheless, a significant error occurred in the estimated hydraulic conditions, suggesting that the forward model has a problem in its ability to reproduce the flow behaviors. Thus, it is necessary to improve the forward model in future studies.