[P-32] An Explainable Artificial Intelligence for Designing Removable Partial Denture Framework
[Abstract]
[Objective]
A well-designed framework of removable partial dentures (RPD) impacts the treatment prognosis. We are developing an explainable AI model to design the framework of RPD using Convolutional Neural Networks (CNN). As the initial step, we developed a model that design the major connectors and examined to make predictions interpretable using Gradient-weighted Class Activation Mapping (Grad-CAM).
[Method]
Data was collected from 1000 RPDs (457 maxillary and 543 mandibular) designed by the specialists of the Japan Prosthodontic Society at Tokyo Medical and Dental University Hospital from 2007 to 2019. Oral examinations (dentition defects, prosthodontic status, and periodontal condition of each tooth, and characteristics of mucosa) were extracted as the input information. Based on a clinical algorithm, the model predicted the denture type (resin/metal), unilateral/bilateral design, presence/absence of the major connector, and types of major connector (maxillary: bar/strap, plate, anterior-posterior bar, horseshoe bar, mandible: bar, plate) from input variables in sequence. In each step, the information predicted in the previous step was also added to the input information. In the CNN model, the input information for each tooth was matrixed, and convolution was performed between adjacent teeth (Python 3.9.8 version). The samples were divided into training, validation, and testing datasets at 64%, 16%, and 20% respectively. The performance of the CNN models was evaluated in terms of accuracy, sensitivity, and specificity. In addition, the Grad-CAM was used to examine which valuables contributed to the predictions.
[Results and Discussion]
The average accuracy of the test data was as follows; denture type: maxillary: 80.4%, mandibular: 74.3%, unilateral/bilateral design: 93.0%, presence/absence of the major connector: 95.0%, maxillary bar/strap: 86.0%, plate: 90.7%, anterior-posterior bar: 100%, horseshoe bar: 84.9%, and mandible: 80.4%, respectively. These results suggest the discrepancy between the predicted and actual case results reflected the special case variations in clinical cases. The Grad-CAM results highlighted the crucial clinical information that contribute to the prediction (Figure), which also make it possible to explain to patients of which factors influenced the predictions.
[Objective]
A well-designed framework of removable partial dentures (RPD) impacts the treatment prognosis. We are developing an explainable AI model to design the framework of RPD using Convolutional Neural Networks (CNN). As the initial step, we developed a model that design the major connectors and examined to make predictions interpretable using Gradient-weighted Class Activation Mapping (Grad-CAM).
[Method]
Data was collected from 1000 RPDs (457 maxillary and 543 mandibular) designed by the specialists of the Japan Prosthodontic Society at Tokyo Medical and Dental University Hospital from 2007 to 2019. Oral examinations (dentition defects, prosthodontic status, and periodontal condition of each tooth, and characteristics of mucosa) were extracted as the input information. Based on a clinical algorithm, the model predicted the denture type (resin/metal), unilateral/bilateral design, presence/absence of the major connector, and types of major connector (maxillary: bar/strap, plate, anterior-posterior bar, horseshoe bar, mandible: bar, plate) from input variables in sequence. In each step, the information predicted in the previous step was also added to the input information. In the CNN model, the input information for each tooth was matrixed, and convolution was performed between adjacent teeth (Python 3.9.8 version). The samples were divided into training, validation, and testing datasets at 64%, 16%, and 20% respectively. The performance of the CNN models was evaluated in terms of accuracy, sensitivity, and specificity. In addition, the Grad-CAM was used to examine which valuables contributed to the predictions.
[Results and Discussion]
The average accuracy of the test data was as follows; denture type: maxillary: 80.4%, mandibular: 74.3%, unilateral/bilateral design: 93.0%, presence/absence of the major connector: 95.0%, maxillary bar/strap: 86.0%, plate: 90.7%, anterior-posterior bar: 100%, horseshoe bar: 84.9%, and mandible: 80.4%, respectively. These results suggest the discrepancy between the predicted and actual case results reflected the special case variations in clinical cases. The Grad-CAM results highlighted the crucial clinical information that contribute to the prediction (Figure), which also make it possible to explain to patients of which factors influenced the predictions.
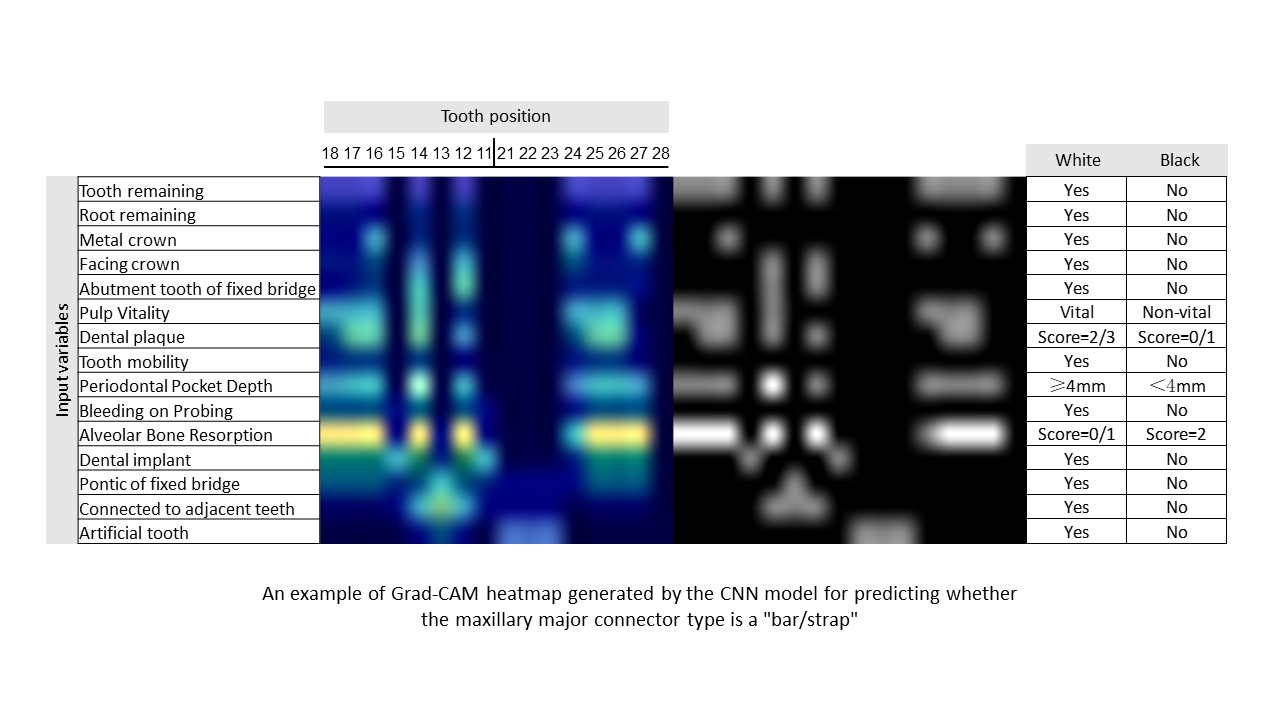