[IL(E)21] The challenge of medical artificial intelligence
【 同時通訳付き】
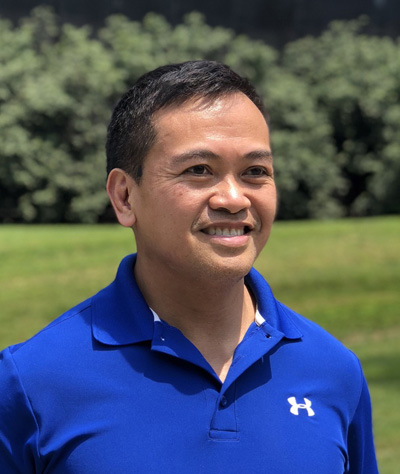
Leo Anthony Celi MD MS MPH has practiced medicine in three continents, giving him broad perspectives in healthcare delivery. As clinical research director and principal research scientist at the MIT Laboratory for Computational Physiology (LCP), and as an attending physician at the Beth Israel Deaconess Medical Center (BIDMC), he brings together clinicians and data scientists to support research using data routinely collected in the process of care. His group built and maintains the public-access Medical Information Mart for Intensive Care (MIMIC) database, which holds clinical data from over 60,000 stays in BIDMC intensive care units (ICU). It is an unparalleled research resource; close to 10,000 investigators from more than 70 countries have free access to the clinical data under a data use agreement. In 2016, LCP partnered with Philips eICU Research Institute to host the eICU database with more than 2 million ICU patients admitted across the United States.
Leo also founded and co-directs Sana, a cross-disciplinary organization based at the Institute for Medical Engineering and Science at MIT, whose objective is to leverage information technology to improve health outcomes in low- and middle-income countries. He is one of the course directors for HST.936 – global health informatics to improve quality of care, and HST.953 – collaborative data science in medicine, both at MIT. He is an editor of the textbook for each course, both released under an open access license. The textbook “Secondary Analysis of Electronic Health Records” came out in October 2016 and was downloaded more the 100,000 times in the first year of publication. The Mandarin translation of the textbook will be released by the end of the year, and a Spanish translation is in the works. The massive open online course HST.936x “Global Health Informatics to Improve Quality of Care” was launched under edX in February 2017. Finally, Leo has spoken in 25 countries about the value of data in improving health outcomes
Medicine presents a particular problem for creating artificial intelligence (AI) because the issues and tasks involved are often neither clearly defined nor black and white. In harsher terms, it is particularly difficult to create ‘artificial’ intelligence when there are still disagreements about concept definitions, what processes are important, and at times, even what outcomes are desirable. Medicine is a surprisingly subjective endeavor whereas valid and useful AI requires not only reliable, unbiased, and extensive data, but also objective (and similarly, unbiased) definitions and objectives. It makes sense that the early successes in AI applications in healthcare are in the field of image recognition. But image recognition in medicine is a low-hanging fruit. Where we need assistance is in the day-to-day complex decision-making that requires data synthesis and integration, tasks we now approach with what is referred to as clinical intuition. This process is notoriously riddled with cognitive biases and typically based on large information gaps, but nonetheless generally accepted as representing the ‘art’ of medicine. Resolving the subjectivity of medicine with the objectivity required for digitization and the secondary creation of AI first involves resolution of a number of questions: What do we want to do? What do we need to do? What can we do?